Three ways AI can enhance radiology imaging workflows – right now
Some observations and proof points of how AI can help address the operational and clinical challenges stifling radiologists’ workflows.
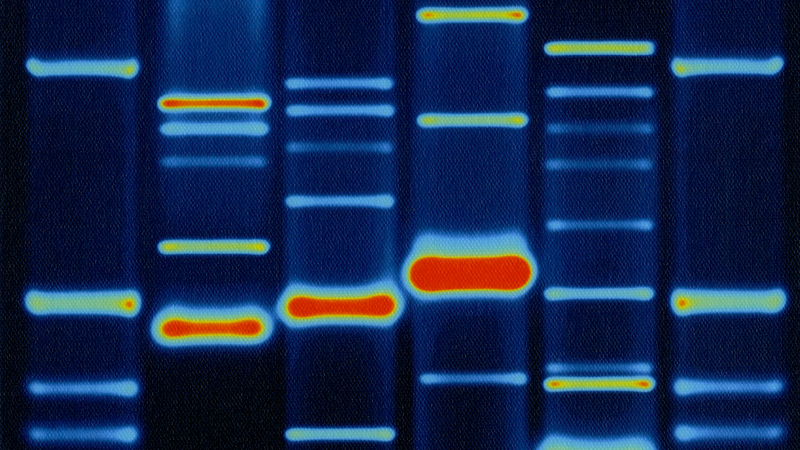

Raj Chopra
Chief Medical Officer, Merge

Marwan Sati
Distinguished Engineer and Vice President, Merge
While the initial driver of AI development in radiology was to improve disease detection, it has become apparent that there are many other areas where AI can help improve workflows and reduce workloads for radiologists. Consider the many workflow steps that comprise radiology: image ordering, scheduling, acquisition, worklist distribution, reading, reporting, patient follow-ups. Each of these steps is an area ripe for targeted AI applications to help improve efficiency and reduce errors for radiologists, technologists, and care teams. Right now, we have a great opportunity to create a radiology ecosystem where every workflow step benefits from new insights and efficiencies that AI can bring to the table.
Think of AI adoption in healthcare as following a similar path of AI in the auto industry. We’re still a long way out from fully driverless cars, but concrete, incremental progress is being made. Take the gradual and specific adoption of automated features in automobiles: blind spot detection, lane detection, front collision detection. These innovations build on each other and pave the way to more innovations and broader adoptions. Now imagine this same approach in radiology: a series of targeted applications that leverage AI to augment the professional.
This kind of intelligent imaging ecosystem, built on a foundation of focused AI applications, is what will help make radiologists more efficient and drive better outcomes for both clinicians and patients – not down the road, but right now.
In this piece, we provide a few examples of how AI can be leveraged to help improve radiology workflow efficiencies today.
1. Intelligent worklist distribution
Dr. Chopra recently conducted his own informal survey of approximately thirty practicing radiologists, and found that around 70% agreed that unequal work distribution and unfair compensation are among the largest sources of job frustration.
To assign imaging studies for retrieval, many PACS rely on individual radiologists’ self-selection of cases to read, or rules-based approaches. This leads to high variability in caseload distribution. At sites where compensation has a “relative value unit” (RVU) component, which assesses and rates studies by their difficulty to read, some radiologists may “cherry pick” studies, choosing the ones with higher compensation or lesser difficulty, consequently leaving other radiologists on the team frustrated. Manual or partly automated caseload distribution can also result in uneven assignments between radiologists, exacerbating burnout and compromising timely, accurate delivery for patient care.
There is a lot of room for improvement in optimizing which studies go to which radiologists based on their specialty and personal preferences. An “intelligent worklist” approach can achieve this by integrating AI to significantly reduce the variability of caseload distribution -- and reduce the friction between radiologists that comes with uneven workloads. Like how the Netflix algorithm leverages your preferred previous shows when suggesting new ones, an AI-driven worklist can consider radiologist preferences alongside other parameters, such as fair RVU distribution, radiologist specialty, urgency, peak times, etc.
In a recent study, presented at the SIIM (Society for Imaging Informatics Management) Conference on Machine Intelligence in Medical Imaging (CMIMI’24) in October, strong evidence showed the power of an intelligent worklist to improve caseload management. (See here for the abstract.) The study compared radiologists’ results working with and without an intelligent worklist. We found that an intelligent worklist approach resulted in a 34% more equitable distribution of studies. By dynamically balancing studies based on priority, value, radiologist preference and specialty, and turnaround time, an intelligent worklist approach can help ensure studies aren’t being assigned on a first come, first serve basis, but rather are matched to the radiologists best equipped to review them. You can learn more by reading the study report, “Enhancing Equitable Study Distribution Using an Automated Worklist Algorithm."
2. Generative AI for reporting workflows
AI boasts potential to increase efficiency in reporting, by integrating AI results into radiology workflows and automate steps in the viewing and reporting activities. AI findings such as measurements can appear as DICOM image annotations within the viewer. Radiologists can then quickly confirm or reject these results and automatically populate structured reports with the quantitative data. This completely sidesteps the grunt work of manually measuring findings and then inputting the data into reports. This is enormously helpful in situations like tracking lesions over time to assess disease progression. A study we performed with deeply integrated AI for lung nodule detection showed a 23% improvement in efficiency over current manual methods for tracking patients with four or more lung nodules. You can learn more by reading the study report, “Reducing Chest CT Interpretation Time Using Advanced Lung Nodule Workflow Functionality."
Generative AI has also shown promise on this front, with the capability to accurately read and understand dictation, convert it to structured text that automatically populates a report, and do so all while accurately capturing the radiologist’s style of reporting. At RSNA 2023 Rad.ai was the first to demonstrate such functionality in their product, demonstrating progress over time. Rad.ai had previously shown automated generation of impressions. Automated AI impressions are now more commonly available through other reporting vendors, including M*Modal, and automated population of dictated prescriptions to structured reports are also being introduced by all the major reporting vendors.
Generative AI is also good at reading and understanding complex radiology and cardiology reporting guidelines. In our research we’ve found that an AI agent for reporting can work in tandem with an AI agent trained in guidelines to help radiologists create more compliant reports.
3. Automated exam follow-up detection
According to the American College of Radiology, up to 10% of radiology reports contain follow-up recommendations – but approximately half of these follow-ups are never acted on by patients. More worryingly, lung nodules represent about half of these missed follow-ups. For patients with incidentally detected pulmonary nodules, more than 60% do not pursue the appropriate follow-up care.
This is an area ripe for improvement – and AI can help fill this gap. Natural language processing tools can be used to identify findings in prior patient imaging reports that call for follow-ups. That information can then be automatically cross-referenced with the physician’s scheduling system to determine whether the patient has been contacted for a follow-up – and if not, can then automatically begin scheduling for follow-up outreach and appointments. This is a relatively narrow and low-touch AI application but one that can carry enormous potential implications for patient care and mortality rates.
Building an intelligent, futureproofed imaging ecosystem
AI can be a valuable tool in delivering patient care faster and more accurately, but always in a way that is complementary to the radiologist’s judgment and expertise, not in place of it. Being a highly digital area of medicine to begin with, radiology doesn’t require radical rebuilding to take full advantage of AI’s potential.
The examples we just discussed show a few ways that targeted AI applications, which are not diagnostic by design, can also help to reshape radiologist workflows into more resilient, intelligent imaging ecosystems. We believe that the industry is just starting to scratch the surface of how AI can transform healthcare, for clinicians and patients.
The takeaway: AI adoption may be slow, but a growing body of proof may soon change that
The healthcare leaders we talk to among our client organizations are open about the fact that they are considering how to evaluate, justify and invest in AI. There are opportunities to start making incremental and valuable changes, but many leaders don’t know where to start. It is usually of interest to learn that AI consulting is a fast-growing area in healthcare IT professional services.
The newly announced Merge Consulting Services division is focusing on AI adoption advisory services among its first practice areas. As more technology transformation professionals and IT consultants get involved in successful AI projects, the knowledge base grows. Organizations that might not have the expertise in-house can now turn to external consultants and advisors to get started. We look forward to sharing some of these success stories in future articles.
Related Articles
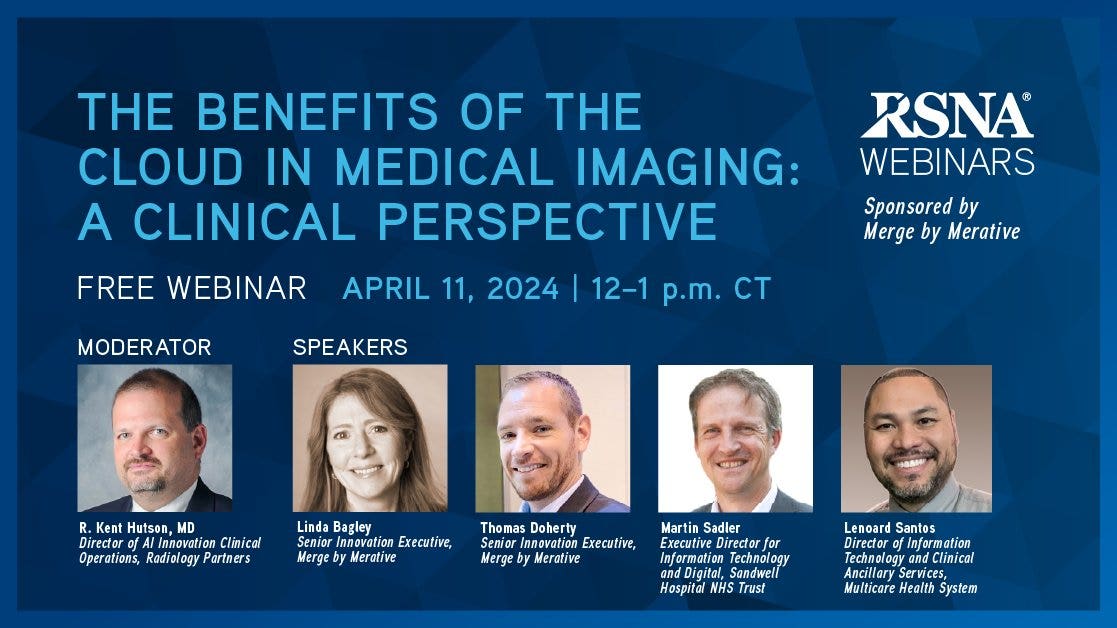
Cloud in medical imaging: 3 takeaways from RSNA webinar on the benefits to radiologists
Merge recently had the great privilege of participating in an RSNA webinar on the...
By Linda Bagley | 5 min. read
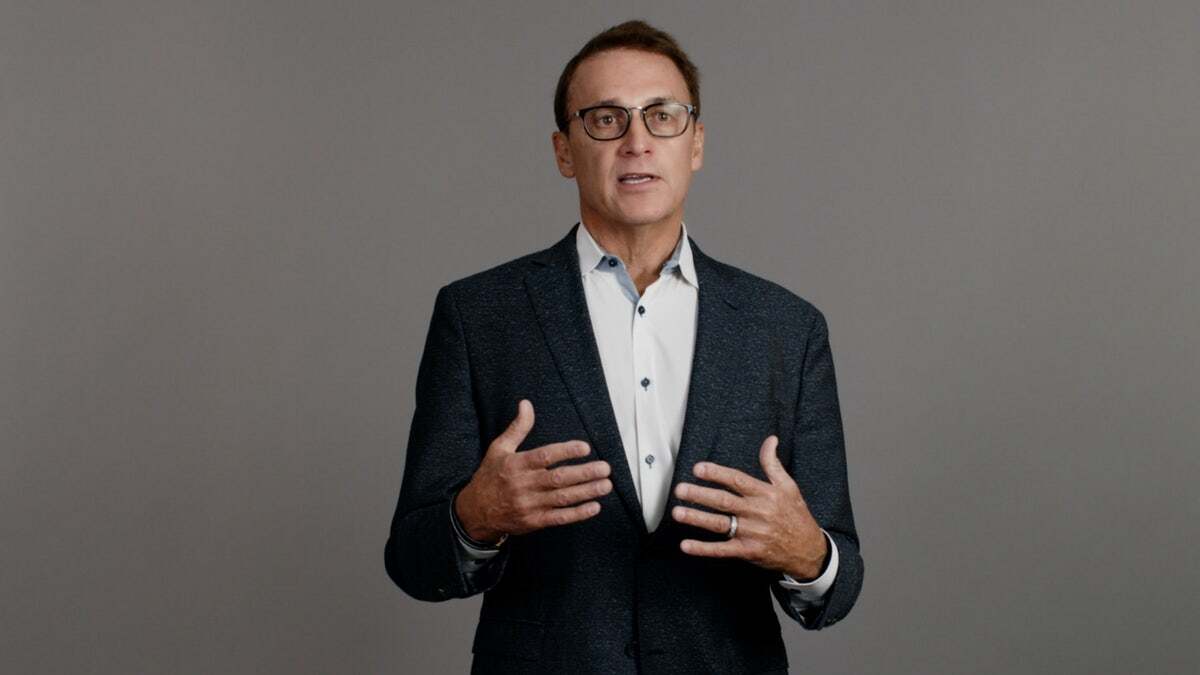
How a new PACS system helped Regional Medical Imaging simplify breast imaging workflows
Dr. Randy Hicks, owner and CEO of Regional Medical Imaging, an imaging service...
By Julie Pekarek | 2 min. read
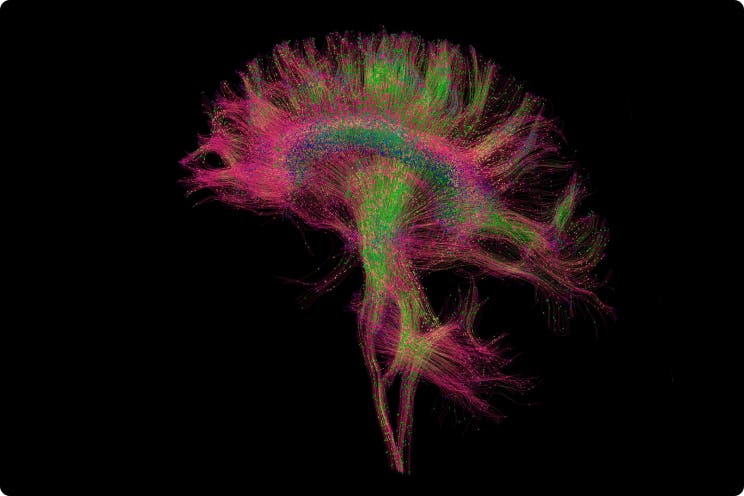
4 Ways this RSNA Panel Laid Out The Future Of Imaging AI
The paths forward to more rapid and widespread AI adoption in medical imaging.
By David Gruen | 6 min. read
Ready for a consultation?
Our team is ready to answer your questions. Let's make smarter health ecosystems, together.