Five questions to ask before choosing a real-world data provider
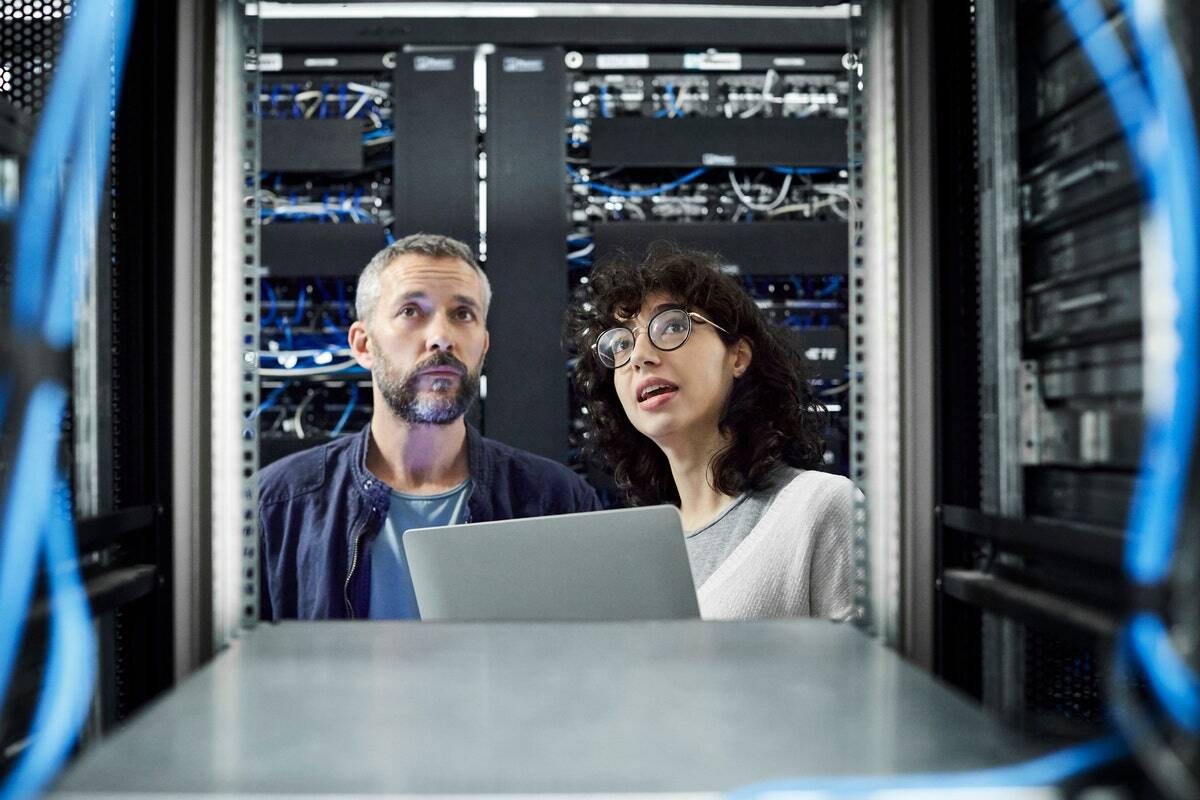
When you’re working with real-world data (RWD) in a clinical trial setting, you must work with what you’ve got. Sometimes, that means settling for data that’s close to, but not exactly, what you want. Other times, the data you really want may be missing from the databases you have. The key to leveraging real-world data effectively is to align your data sources with your trial design and research questions. Finding the right data begins with finding the right data partner.
New podcast: Liisa’s perspectives on finding balance with RWD
There are several important considerations that come into play when selecting a data partner. The breadth and quality of data is obviously one of those considerations. Understanding and adhering to appropriate data governance and being able to convert data into real-world evidence (RWE) are other factors that warrant attention. When working with trial sponsors and contract research organizations (CROs), I find that making the right decision about data sources is the result of asking the right questions.
What characteristics matter most
Where does the data come from?
To say the US healthcare system is complex would be an understatement. There are multiple touchpoints in the life of a patient: physicians, hospitals, specialized treatment centers, insurance companies, pharmacies, self-insured employers and the list goes on. Each touchpoint generates its own unique data, which can range from data that is highly detailed but narrow in scope (e.g., clinical centers) to data that is exceptionally broad, but with some limitations (e.g., insurers, self-insured employers). In addition, new technologies such as wearable devices represent an expanding source of RWD.
For clinical trial development, patient data that covers a long period of time (something we call a longitudinal view) and represents a wide range of geographies can be especially helpful in designing and optimizing trials for success. As a standalone data source, administrative claims data from self-insured employers offers one of the widest possible views of a US-based patient. Since the employer is ultimately responsible for paying all medical costs, it sees everything: prescriptions, physician visits, urgent care visits, etc. The MarketScan Research Databases are some of the few examples of a closed administrative claims database, meaning that the healthcare data captured includes all healthcare interactions regardless of provider type, clinical support tools, or billing systems.
See how longitudinal RWD is helping CHU Sainte-Justine safely and effectively study the associations of medications with pregnancy outcomes before, during, and after pregnancy. Read case study
Who does the data represent?
Increasingly, CROs are being tasked with ensuring that their trials fairly represent a broad socio-economic and ethnic population. The FDA has issued regulatory guidance calling for “meaningful representation of racial and ethnic minorities in clinical trials,”1 which can be a challenge depending on the data source. For example, if relying exclusively on data from a regional health plan, the data may be insufficient if attempting to reflect the race/ethnicity of those with a disease nationally. Therefore, data aiming to be representative of the U.S. must also capture data across a variety of health plans, policies, and insurance firms.
Prioritizing diversity in data collection minimizes the risk of bias and provides researchers with additional variables to better understand your population's health trends. Having access to a more holistic picture of healthcare drastically enhances the quality of research outcomes. For example, when conducting HEOR studies, the ability to combine actual cost data with diverse, representative data leads to more robust studies. For epidemiology studies, representativeness is critical in collecting accurate measures of incidence and prevalence rates.
How are we going to use this data?
When analyzing clinical trial data, researchers are evaluating data that was collected with an explicit purpose for that specific clinical trial. When integrating real-world data into clinical development, researchers are taking data that was collected for one purpose and using it for another. The reasons why data was collected can impact which variables are available and/or regularly populated. For example, administrative claims are collected for billing and insurance reimbursement purposes, so financial metrics will be robust; clinical details like vital signs may be included only opportunistically.
Social and environmental constructs are also important to consider with real-world data. If access to healthcare services such as specialist care is problematic for certain patient populations, then analyses using information about specialist care will need to be interpreted with that context in mind. Data coming from wearables can fluctuate based on personal and environmental factors. The accuracy of early pedometers, for example, was susceptible to positioning and speed; even today, the activity type can impact the validity of these types of data. The phrase “fit for purpose" is often used when talking about data and its use. Making sure that real-world data use and subsequent interpretations are made understanding that “fit for a purpose” doesn’t mean “fit for all purposes” is key to appropriate use.
Can this data be combined with other sources?
CROs frequently will draw from multiple real-world data sources to support their clinical trials. Ensuring that data is linked or stacked according to governing data use agreements in addition to making sure that personally identifiable information (PII) is protected is paramount. To effectively share data without compromising confidentiality, real-world data providers may use tokenization as a means of linking patient identities without revealing any PII. Tokenization allows CROs to extend the clinical trial scope by, for example, connecting clinical data from cancer centers with administrative claims data to track patient experiences post-treatment.
See linked claims + EHR database
Do we have the skills to manage and analyze this data?
Many CROs have very talented analysts and researchers in their ranks, but they may lack experience in dealing with the nuances of large, real-world data. By definition, clinical trial databases have strict parameters. And real-world data can be just like the real world, messy. Understanding how to execute a robust analysis while using scientifically acceptable data transformations can be a new challenge for CROs that may require external support from the data provider. Clinical trial data may have missing assessments or null values. Depending on the source, real-world data can have temporal gaps, missing values or values outside of reasonable parameters; often, all of these are present. Selecting a data provider that also offers analytics services and insights into their data can greatly reduce the complexity of analyzing real-world data down the road.
As you can see, selecting a real-world data provider involves considerable thought and study. The investment you make in researching your data provider has its own payoff, however, as the FDA and other agencies are increasingly looking to clinical trial developers to justify their data decisions. This means not only being able to explain why a particular data source was selected, but also justifying those sources that were excluded. With so much on the line, making the right choice has never been more important.
Sources
- “FDA takes important steps to increase racial and ethnic diversity in clinical trials,” fda.gov, April 13, 2022, https://www.prnewswire.com/news-releases/fda-takes-important-steps-to-increase-racial-and-ethnic-diversity-in-clinical-trials-301524987.html
Related Articles
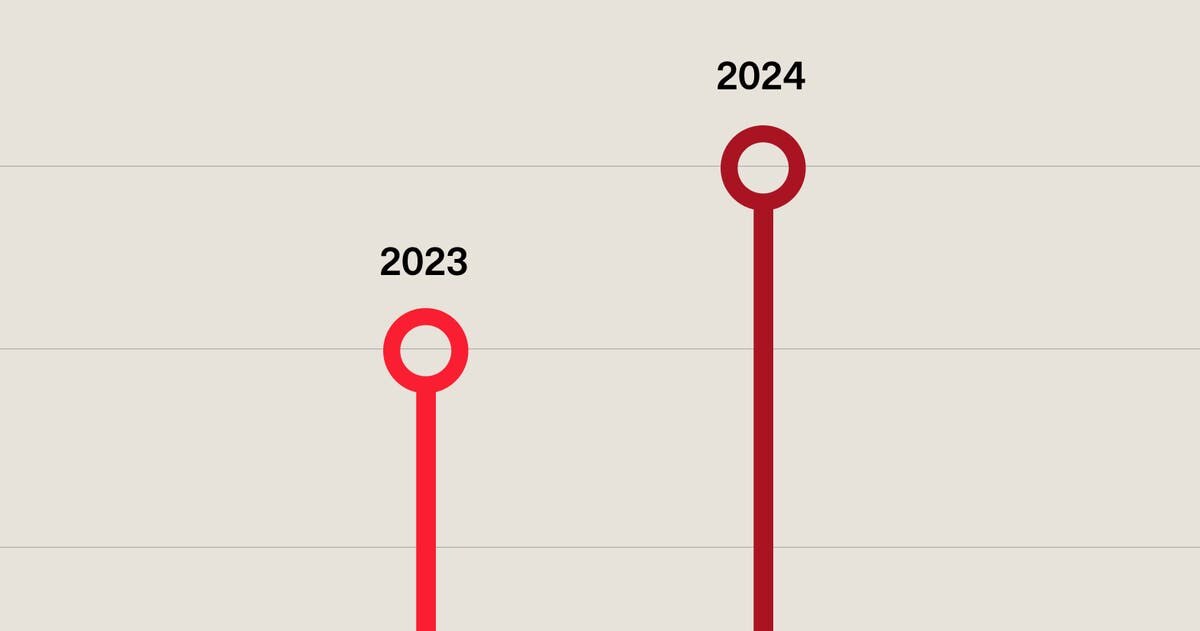
Building the next generation of MarketScan: A year in review and what’s in store
About a year and a half ago, we said goodbye to IBM Watson Health and set off on an...
By Merative | 4 min. read
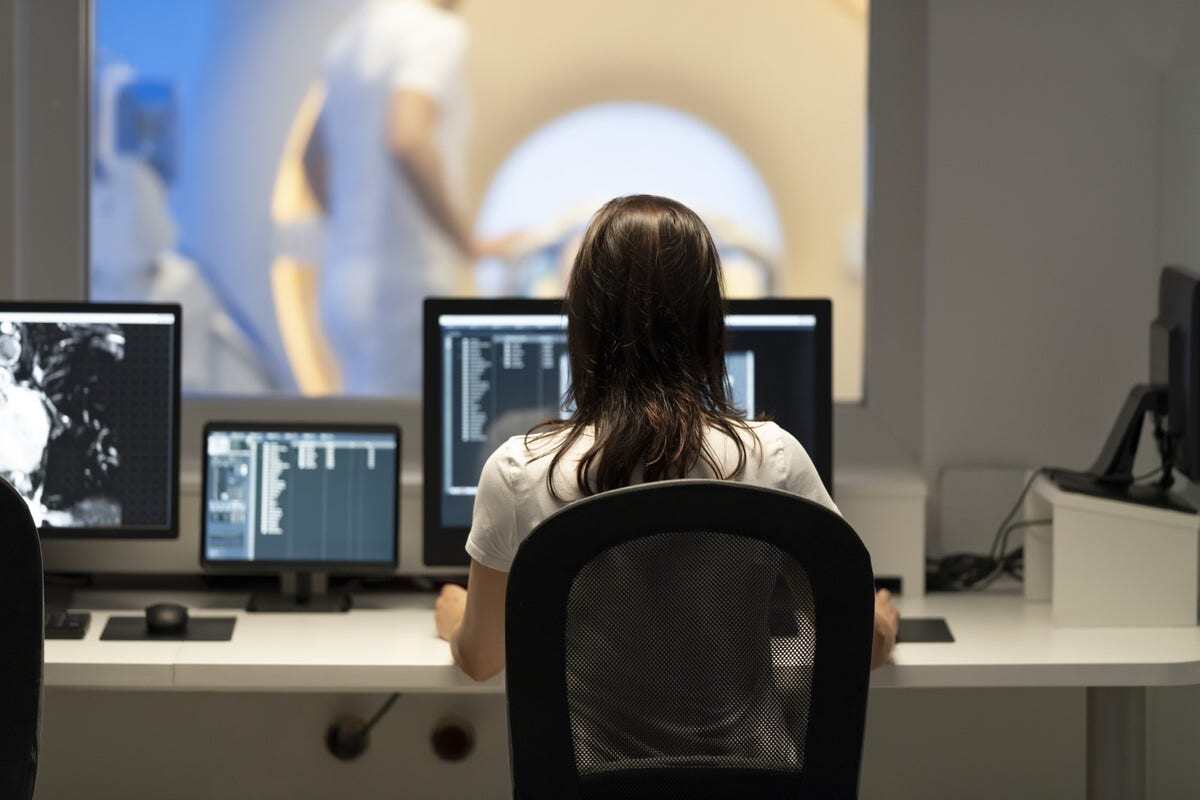
Is real-world data the real deal?
Real-world data is Liisa’s world. After receiving her PhD in health policy and...
By Liisa Palmer | 4 min. read
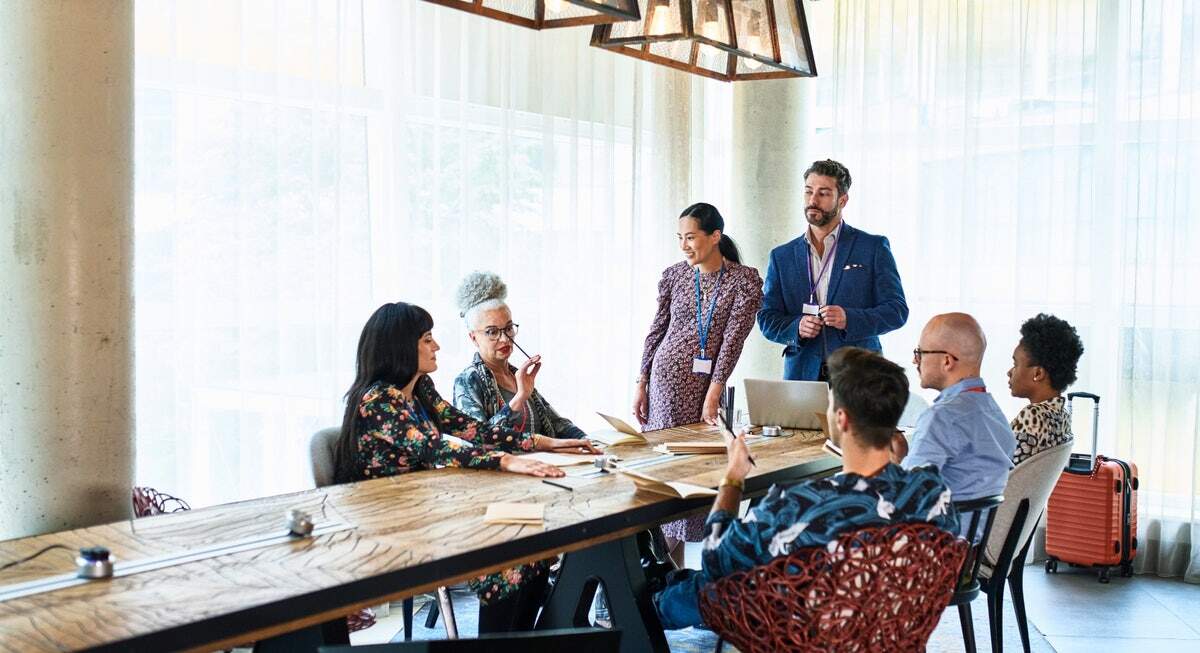
Optimize sample size and observation time: A case for employer-sourced administrative claims data
Closed administrative claims databases capture the complete range of healthcare...
By Merative | 5 min. read
Ready for a consultation?
Our team is ready to answer your questions. Let's make smarter health ecosystems, together.