Unlocking real-time insights: how on-demand analytics reduces latency for faster decision-making
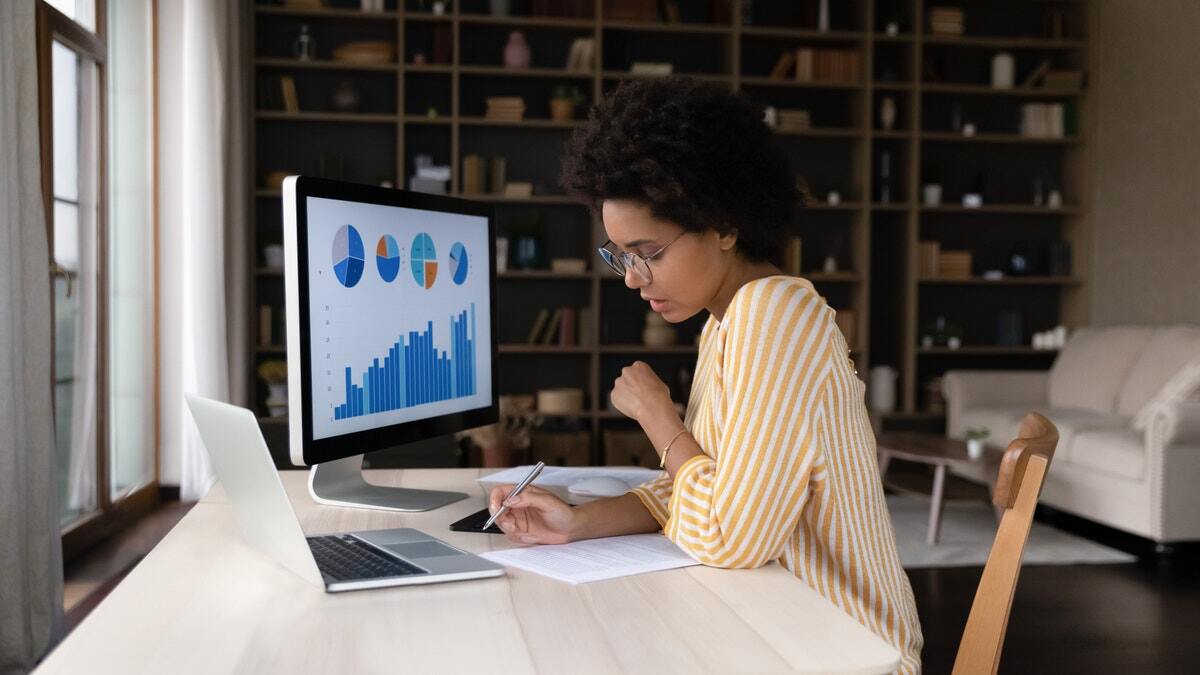
When it comes to data-driven decision making, analytic latency can have a major impact on an organization’s ability to make fast decisions and prioritize member outreach correctly and efficiently. This will ultimately reduce organizations’ ability to create proactive strategies for improving population health, while also controlling costs. While it is critical for payers to address data latency in their processes, it is equally important to consider analytic latency in data-driven initiatives. It is not enough for the data to be available; it needs to be actionable.
- Data latency is the time between the creation of data in source systems (e.g., claims, enrollment, network management) and the time at which the same raw data is available in the enterprise data warehouse (EDW) for reporting.
- Analytic latency is the time between the availability of the raw data in the EDW and the time at which that data is transformed into actionable analytics (e.g., risk scores, gaps in care, episodes of care, outpatient events, inpatient stays).
Just 5% of patients account for 50% of total costs, so the need to identify at risk patients, their conditions, and their risk drivers is critical. Pairing the right models with the right cadence can make data-driven decisions more effective. The ability to reduce analytic latency and run analytics on demand (as you need them, i.e., nightly, weekly, etc.) can reduce time to insights that have wide impacts on strategies relying on those insights. Timeliness of insights impacts the ability to intervene for patients with gaps in care that may otherwise be resolved, reducing critical events and exacerbations of conditions down the line.
Lagging insights on care gaps may also reduce performance ratings, such as Star ratings for Medicare Advantage plans, HEDIS, and also impact value-based care arrangements. Timely insights allow payers and employers to identify newly diagnosed conditions as data becomes available for program enrollment and increases the ability to properly quantify patient risk for stratification and prioritization. Episodic costs may also be understated due to analytic lag. Where the ability to run analytics on demand may be most notable is in predictive analytics models often utilized by care teams to better focus their limited resources and ideally prevent healthcare complications that may have otherwise been prevented.
Impact of analytic latency
A person's health status is dynamic, and advanced predictive models are sensitive to data variations, particularly for models intended for outreach and prevention. As an example, let’s look at avoidable admissions. Avoidable admissions identify hospital admissions that could have potentially been prevented through high-quality outpatient care. Avoidable admissions are often measured using AHRQ’s Prevention Quality Indicators (PQIs). The impact of avoidable admissions will vary by methodology employed, the population being assessed, and the conditions being evaluated. AHRQ’s recent analysis shows 3.5 million potentially preventable adult inpatient stays accounted for $33.7 billion in aggregate costs.
To highlight the potential financial impact of a reduction in avoidable admissions for four high-prevalence chronic conditions, we utilized Merative’s MarketScan Research Databases using AHRQ criteria. Reducing the number of avoidable admissions by just 10% would result in more than $1M in savings per year for a 250,000-member plan or $0.33 PMPM.1
Care managers need to prioritize patients for outreach and intervention, so having access to critical information as it becomes available is necessary. Models such as the Risk of Hospitalization rely on several key inputs for scoring and stratifying patients for intervention, so capturing timely changes to those inputs has implications for scoring outputs. This can include recent changes to disease history such as new diagnoses or variations in disease severity, prescription drug use to treat chronic conditions, utilization inputs such as visit counts, capture of specific procedures, patient cost, and visit recency. Prioritization will change as inputs change.
The clinical specificity of the Risk of Hospitalization model also helps inform care managers of the drivers associated with patient risk. After stratification, the model can inform care coordinators of the specific drivers of risk, allowing them to tailor their interventions to the patient.
Outreach alone, however, is not enough if care managers are unable to reach members within a critical time frame. Predictive analytics such as the Risk of Hospitalization model are used so that care managers can take a proactive rather than reactive approach. A proactive approach aims to prevent hospitalization events in the first place as well as potential readmissions rather than manage patients after the fact. With a predictive window of 6 months, reducing analytic latency is critical to the ROH model and models like it. Intervention strategies can also vary based on short- and long-term interventions so reducing analytic latency allows for better prioritization along with a more comprehensive approach.
Conclusion
Near real-time analytics are needed for workflows, value-based care, and population health initiatives. Use cases will vary, but the ability to transform data into predictive, prescriptive, and actionable analytics when you need them is a critical need for payers. While payers have made advancements to address data latency in their processes, analytic latency is another strategic imperative to focus on, directly affecting data driven decisions. The ability to run analytics when you need them improves payers’ ability to advance population health, close gaps in care, increase performance ratings, and will ultimately impact their bottom-line.
Learn more about running analytics on demand with Truven Flexible Analytics.
Sources
-
Data based on MarketScan continuously enrolled Commercial population under 65, April 2022-March 2023 rescaled to a population of 250,000 members.
Related Articles
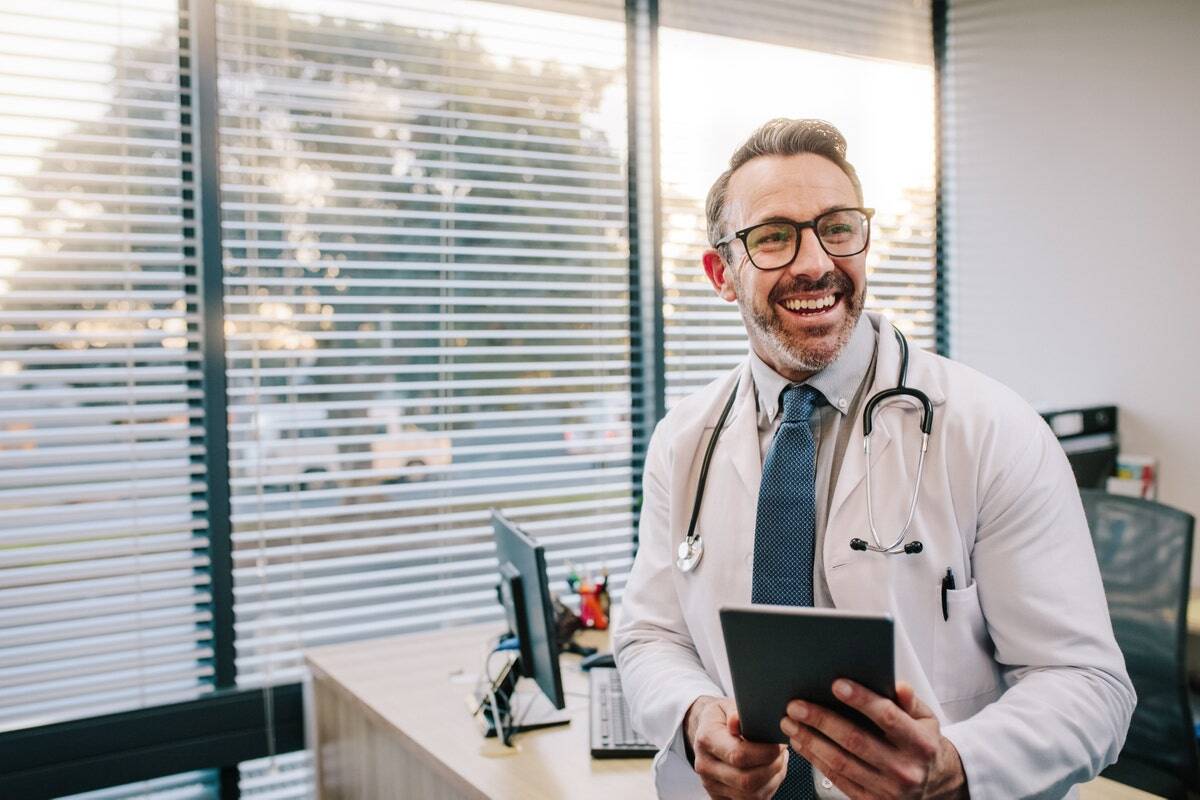
Optimizing value-based healthcare: 5 strategies for effective provider network management
In today's rapidly evolving American healthcare industry, the collaboration between...
By Brandi Hodor | 6 min. read
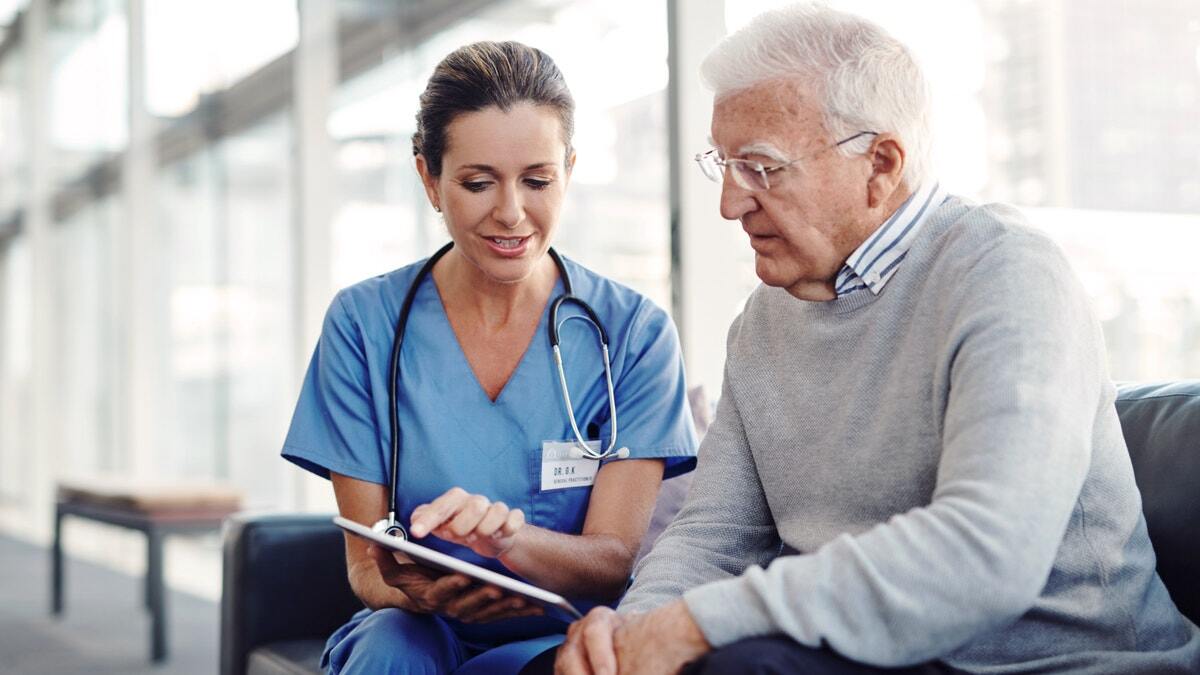
Healthcare analytics to identify the right member, the right intervention, at the right time
Managing the health of your member population is an important responsibility, but...
By Carrie Frederick | 5 min. read
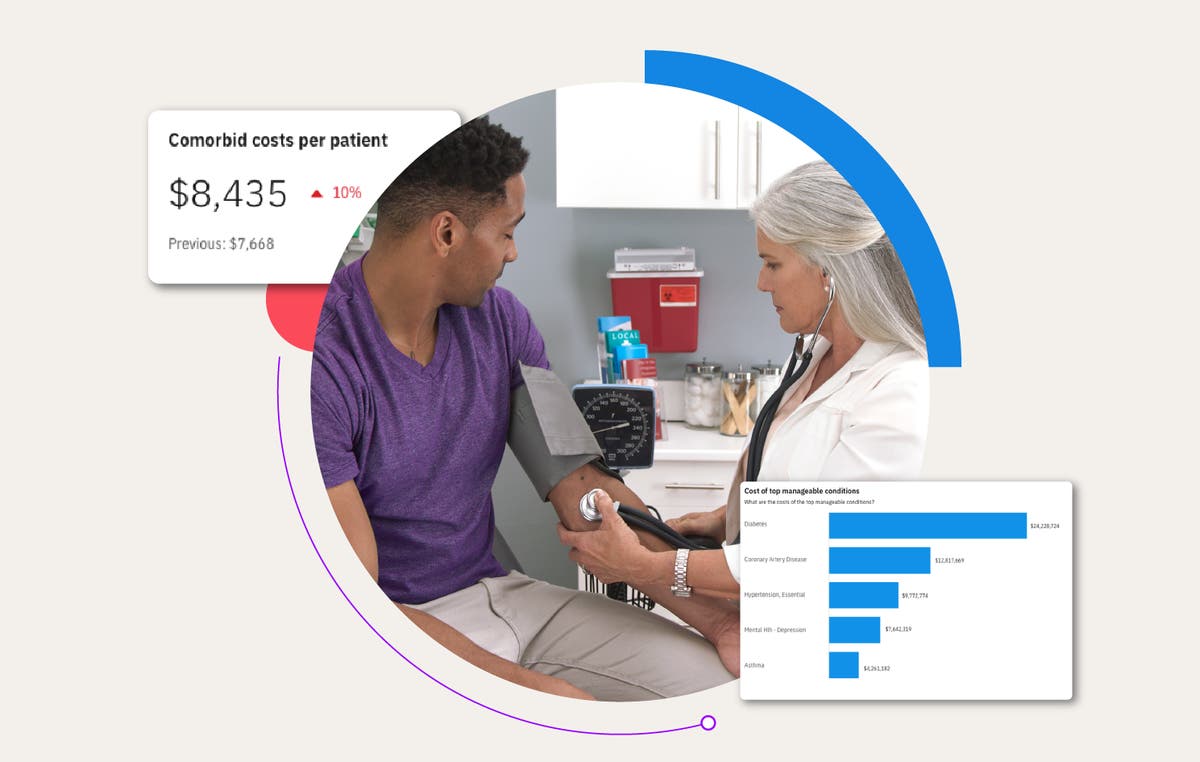
How healthcare analytics help employers reduce costs and improve employee health
Year after year, employers grapple with rising healthcare costs that feel beyond...
By Jennifer Huyck | 4 min. read
Ready for a consultation?
Our team is ready to answer your questions. Let's make smarter health ecosystems, together.